About Professor Emma Lundberg
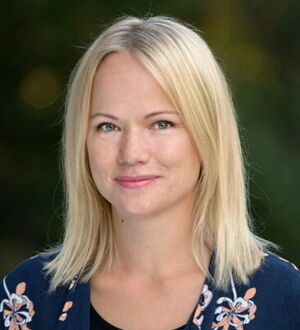
Emma Lundberg, Professor in cell biology proteomics at KTH Royal Institute of Technology, Sweden
She is also the director of the Cell Atlas, an integral part of the Swedish-based Human Protein Atlas (HPA) program, an open-source resource for human proteome exploration. As part of the HPA, the Cell Atlas project provides high-resolution images of the expression and spatio-temporal distribution of RNA and proteins in human cell lines. Prof. Lundberg was the recipient of the annual prize (2017) of the Swedish National Association of Chemical Engineers (SKR), awarded for her innovative research in mapping the human proteome. She is also a Wallenberg Academy Fellow (category technology, 2017-2021).
Could you please tell us a little bit about your current research?
Prof. Lundberg: I am the director of the Human Protein Cell Atlas (HPA) project, which involves mapping the distribution of human proteins in cells. As part of this large-scale project, we generate lots of high-resolution confocal images with the goal to interpret the patterns and distribution of proteins in cells. This is not a trivial task, since about half of all human proteins are found in two or more cellular compartments – so, it is often a mixture of two different patterns. We are also working with dozens of different cell lines, which have different morphology and present their organelles slightly differently. When we started this project, we did all the annotations manually and then, we gradually built machine learning models to classify the protein distribution patterns.
How does the use of artificial intelligence and machine learning in microscopy fit into your research, and what benefits does it provide?
Prof. Lundberg: Using machine learning models saves us a lot of time, but I believe that the real wins will come when we develop models that can embed spatial information into a format that we can integrate with other types of molecular measurements, such as proteomics data or single-cell sequencing data. Another major benefit of machine learning algorithms is more unbiased image analysis. For example, it could help us find cells that are very similar to each other, simply by their visual appearance, or we can perform anomaly detection to find rare cells.
As part of the HPA, we have mapped the subcellular distribution of the majority of human proteins and now, we are starting to look at the temporal dynamics and proteins that tend to show variation in their distribution between neighboring cells. For this, we need robust models that can quantify spatial patterns at the single-cell levels, to help us understand subtle changes in cellular morphology. Biological processes are driven by the interaction of certain proteins, and small spatial rearrangements may have very significant functional consequences, so we want to be able to identify and quantify these subtle differences.
When it comes to real-time image analysis, I hope that new microscopes will provide opportunities to augment our view – to help us predict for example the cell cycle position of the cells, or which cells are going into apoptosis, or predict a membrane label and the DAPI stain from bright-field so that we can add all these augmentations to our microscope view in real-time. I hope that we will end up with some kind of closed loop between data-driven image acquisition and AI-driven image analysis in the end, as well.
Can you please tell us a little bit more about the benefits of being able to analyze data while you are acquiring your images?
Prof. Lundberg: As part of the HPA project, we sometimes examine rare events, such as specific enzymes that translocate into the nucleus in rare cells, where their function is unknown. The use of near real-time analysis during imaging is necessary when we are examining these rare, spatial reorganizations in the cell. This way, we can specifically adopt the image to the event, particularly when we are studying dynamic events. When using frequent time-lapse imaging, this method also allows us to do sparser imaging to avoid phototoxicity, and when we detect the rare event that we want to study, we can selectively image that cell.
In contrast, if we were analyzing these rare events using manual image analysis, we would need to stop the experiment, perform the analysis, and then repeat the experiment.
Since you are developing these technologies yourself, are you using them to acquire real-time results?
Prof. Lundberg: We have light-weight models that can run in real-time. However, we have not closed the loop and finished that integration yet, but we hope to do so in the near future. For example, we have developed a model that can predict a membrane stain from bright-field images and with it, we can achieve segmentation of cells with high accuracy in real time. This means that we can measure the fluorescence in segmented cells in real-time, during microscopy.
What is the main value that artificial intelligence and machine learning could bring to everyday microscopy?
Prof. Lundberg: AI-powered analysis could optimize and streamline microscopy image processing, so that you can improve your signal-to-noise ratios, deconvolve images or make them super-resolved. It has tremendous potential in improving image quality, revolutionizing microscopy.
In terms of analysis, machine learning algorithms could create completely new opportunities for analyzing large data sets. Using modern microscopy, we can generate such big microscope data sets, while AI-assisted analysis makes us well equipped to efficiently and accurately analyze them.
Otherwise, I think that when it comes to imaging, AI is very useful for segmentation, which is a common method applied in image analysis. A good segmentation will take you far, helping you get better quantitative data and you can perform robust statistical analysis. AI-powered does not need to be intimidating – it can range from general models, such as cell segmentation, to advanced algorithms. Most biologists would likely find machine learning algorithms quite useful in image analysis, and there is no reason not to give it a try.
Can researchers who do not work with very large image data sets still benefit from AI-assisted analysis?
Prof. Lundberg: Absolutely. I would say that cell segmentation would be one of the available tools they definitely benefit from. Machine learning algorithms can also be of great assistance in pattern recognition – for example, in identifying specific organelles without markers.
In the future, machine learning models can be used to mine open image repositories. This will also enable opportunities to analyze our own imaging data in relation to existing data. So, even if you generate a small dataset – you can see, for example, if your phenotype matches another phenotype in a database.
Are there any current challenges to applying AI to image analysis in microscopy?
Prof. Lundberg: Yes, of course. There are the challenges of sharing and managing massive image data sets, as well as the computational demands for training the AI models as well as development of easy-to-use interfaces for researchers who are not used to AI models. There can be many hurdles, depending on your area of expertise. If you are an AI expert, you will likely want to develop an even better model, and if you are a microscopist who is not used to using AI models, you may want to learn how to use them. I think there are many aspects related to using machine learning algorithms that can be improved.
Do you think there is resistance from researchers to adopt AI-assisted analysis?
Prof. Lundberg: Overall, I think the imaging community has adopted AI-based tool very well. I think that some resistance is normal, since this is always the case when new analysis tools come along. Iterative tools such as AI-assisted image segmentation, having the user in the loop to manually correct and interacting with the model during training, can help researchers gain confidence in the model because they can see how it gradually improves. But of course, one has to be aware that models are trained on data, and if you input wrong data, the model might give you something that is not reliable as output. Of course, you should not trust AI-powered analysis blindly.
Do you think that supervised machine learning could lower the adoption barrier of AI-assisted image analysis?
Prof. Lundberg: Of course. Typically, supervised machine learning requires some kind of annotation of the data, and that is often what we are used to doing in imaging. For example, when you want to label your cells or mark out regions of interest in your histopathology slides – the generation of this ground proof often creates a bottleneck. Once you have enough ground truth data, you can train good AI models, but you still need experts to generate that ground data. In turn, the quality of the ground truth will determine the quality of your models. I believe that by closing that loop and performing iterative analysis, we can train better models with the guidance of experts to improve their weak spots. This is also a way forward to help the true biological experts gain confidence in a model, so they see how it can improve over time. Having this “human in the loop” approach is an interesting way forward.
What do you think will be the future of image analysis?
Prof. Lundberg: I think that AI models will really help us to understand and mine all the richness of image data. What’s more, AI could help us make image acquisition schemes smarter, so that we can collect better data. AI tools will help us to super-resolve, restore and process the images – producing faster, smarter and higher-quality data and providing new possibilities for interpreting image data, and making predictions from image data.
What limitations do we still need to overcome to see computational analysis becoming fully adopted?
Prof. Lundberg: I think that one of the big limitations is the lack of free public open image repositories as well as a model zoo for sharing AI models for microscopy. If we could share all AI models developed for microscopy as well as access all the already acquired images, imagine all the types of models you could train and the scientific questions you could address!
What would you say to a fellow researcher to get them excited and consider using AI- powered analysis?
Prof. Lundberg: The time is right – there are many good tools available out there, and even if you are not an expert, you can learn how to use them. There is no reason to be hesitant to adopt AI-powered analysis because it can provide you better images and better image analysis. Most microscopists invest a lot of time in planning and setting up their experiments. So, why not invest some time in adopting this new technology to improve your analysis as well?
If you would like to learn about AI-powered image analysis, there is a very friendly and helpful community out there. There are also many open-source tools and forums on the topic, so there is no reason not to go for it.
Currently, in the HPA database, we have about 10 million images and over 1.2 million users per year. So far, we have only allowed users to download data from us. Maybe, at some point in the future, we will be able to allow users to upload their own image data and query our database with an image, in addition to words. This is a very tangible goal, and I believe that AI presents many such opportunities.